Our Technology
Measuring, predicting, and engineering protein-protein interactions
Our Experimental Platform
AlphaSeq
We use synthetic biology to generate high-resolution protein binding data at scale.
Vastly Multiplexed
Characterize whole protein-protein interaction networks with millions of interactions
Highly Quantitative
Measure physiologically relevant interaction strengths spanning picomolar to micromolar affinities
Widely Versatile
Antibody discovery, molecular glue target discovery, affinity tuning, protein interface engineering, and more
Our Computational Platform
AlphaBind
We apply machine learning (ML) to accelerate discoveries and engineer better therapeutics.
Predict Affinities
Learn from over 750 million affinity measurements to predict binding strength from protein sequence
Capture Diversity
Explore a massive sequence space around the parental by learning the effects of stacked mutations
Iterate Rapidly
Cycle from model-proposed sequences to binding data in six weeks to quickly validate and refine models
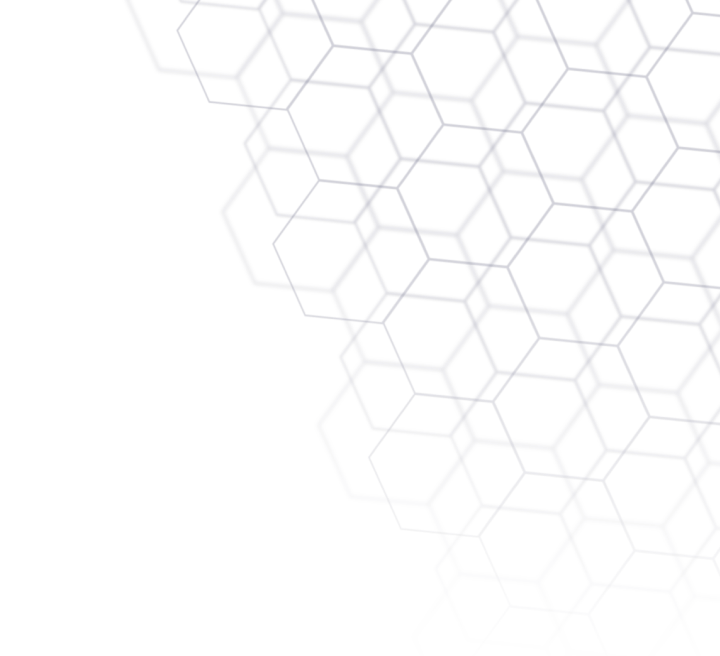
Our PROCESS
AlphaSeq + AlphaBind Explained
- AlphaSeq
BUILD
Two yeast surface display libraries are built from synthesized or template DNA. Displayed proteins may include antibodies, cell surface receptors, intracellular proteins, bacterial or viral antigens, peptides, and more. The libraries are mixed in a liquid culture, allowing A and Alpha cells to collide. FUSE
As cells collide, proteins on the cells’ surfaces may lead them to bind, where the probability of binding depends on protein interaction strength. Each surface expressed protein is paired with multiple unique DNA barcodes. Bound A and Alpha cells fuse, combining DNA barcodes into a single fused cell. A recombination event then pairs the barcodes onto one strand of DNA.MEASURE
Paired A and Alpha barcodes are read and counted with next generation sequencing. Stronger protein-protein interactions result in a greater frequency of observed barcode pairs. Protein-protein interaction affinities are computed using internal controls and barcode replicates.
- AlphaBind
COMPILE
Data from each new AlphaSeq experiment is added to a growing database of hundreds of millions of protein-protein interaction affinity measurements, including negative data. Control interactions are added to each assay to normalize results between different experiments. TRAIN
Interaction measurements from the database are used to train machine learning models that relate protein sequence to binding affinity. ML is particularly powerful for teasing apart nonlinear effects from combining mutations, enabling accurate predictions of sequences far from any that have been experimentally tested.DESIGN
Once trained, ML models are used to predict diverse sequences that have desired binding properties (affinities, specificities, cross-reactivities, epitopes, etc.). Computational screens for expression, stability, solubility, and other properties are then used to enrich designs for those most likely to be developable.Validate & REFINE
Model predicted protein sequences are synthesized and tested experimentally with AlphaSeq and orthogonal assays. A <6-week AlphaSeq + AlphaBind iteration cycle enables rapid evaluation and improvement of model performance.
Our focus areas
Applying AlphaSeq + AlphaBind
Our main areas of focus are listed below. Please contact us to inquire about these or any other applications of interest.
Protein-protein interactions are central to all of biology and are the key mechanism of action for antibodies, molecular glues, cell therapies, vaccines, diagnostics, and more. Our main areas of focus are listed below. Please contact us to collaborate on these or other applications of interest.
ANTIBODY DISCOVERY and OPTIMIZATION
An antibody’s therapeutic properties are driven by protein-protein interactions, making AlphaSeq + AlphaBind invaluable for finding and optimizing antibodies with a given affinity, specificity, cross-reactivity, and/or epitope. With AlphaSeq, we discover antibodies with the right binding properties, even when poorly represented in a large library. Combined with AlphaBind, we optimize antibodies rapidly for multiple binding properties and bias libraries to also improve developability properties and increase sequence diversity. We apply our antibody discovery and optimization workflow to build our therapeutic pipeline and to partner with industry leading companies and institutions.MOLECULAR GLUE TARGET DISCOVERY
The throughput and sensitivity of AlphaSeq makes the platform well suited to uncover novel targets for molecular glues by discovering and characterizing weak interactions between E3 ubiquitin ligases (or other effector proteins) and target proteins that may be enhanced by a small molecule binding at the interface. We measure interactions between our proprietary E3 ligase library and undruggable target proteins to provide a starting point for the rational discovery of molecular glues. We have discovered and validated many pairs internally and look to partner with industry leaders to progress protein-protein binding insights into small molecule drugs.ML MODEL Development
Our data accumulates in a rapidly growing database with hundreds of millions of quantitative protein-protein interaction measurements, including critically important negative data. AlphaSeq data is both quantitative and multi-dimensional, making it ideal for training highly predictive machine learning models for therapeutically critical properties that include affinity, specificity, cross-reactivity, and epitope. Models are used to fuel internal campaigns at A-Alpha and in partnership with industry leaders.
Select Publications and Posters
- Learn More2024
BioRxiv: AlphaBind, a Domain-Specific Model to Predict and Optimize Antibody-Antigen Binding Affinity
Aditya Agarwal, James Harrang, David Noble, Kerry McGowan, Adrian Lange, Emily Engelhart, Miranda Lahman, Jeffrey Adamo, Xin Yu, Oliver Serang, Kyle Minch, Kimberly Wellman, David Younger, Randolph Lopez, Ryan Emerson - Learn More2024
SITC 2024 Poster: Preclinical Development of Cis-Acting, Affinity Tuned IL-21 Immunocytokines for Cancer Immunotherapy
Ruchi Bansal, Shyam Gandhi, Kenny Herbst, Kerry McGowan, David Noble, Joseph Harman, Jeff Adamo, Leah Homad, Dasha Krayushkina, Carla Talbaux, Jessica Fint, Davis Goodnight, Ryan Swanson, Randolph Lopez - Learn More2024
A High-Throughput Approach for Discovering Glueable Ligase-Target Interactions
Phil Burke, Arpita Sen, David Noble, Ruchi Bansal, Dasha Krayushkina, Kerry McGowan, Natasha Seelam Murakowska, Paxton Reed, Juliana Barrett, Randolph Lopez - Learn more2023
Nature Communications: Machine learning optimization of candidate antibody yields highly diverse sub-nanomolar affinity antibody libraries
Lin Li, Esther Gupta, John Spaeth, Leslie Shing, Rafael Jaimes, Emily Engelhart, Randolph Lopez, Rajmonda Caceres, Tristan Bepler, Matthew Walsh - Learn more2023
AACR 2023 Poster: Cytokine affinity tuning using the AlphaSeq platform to generate targeted immuno-oncology therapeutics
Davis Goodnight, Jeff Adamo, Kyle Minch, Emily Engelhart, Ryan Swanson, Randolph Lopez - Learn more2023
PEGS 2023 Poster: TCR mimetic antibody discovery, characterization, and optimization enabled by the AlphaSeq platform
Emily Engelhart, Davis Goodnight, Randolph Lopez, David Younger - Learn more2023
PEGS 2023 Poster: Machine learning-guided antibody optimization of a SARS-CoV-2 binding antibody enabled by AlphaSeq
Emily Engelhart, Christof Angermueller, Ben Jester, Zelda Mariet, Ryan Emerson, Davis Goodnight, Kyle Minch, Kim Wellman, Randolph Lopez, Mike Frumkin, Lucy Colwell, David Younger - Learn more2022
Antibody Therapeutics: Massively multiplexed affinity characterization of therapeutic antibodies against SARS-CoV-2 variants
Emily Engelhart, Randolph Lopez, Ryan Emerson, Charles Lin, Colleen Shikany, Daniel Guion, Mary Kelley, David Younger - Learn more2017
PNAS: High-throughput characterization of protein–protein interactions by reprogramming yeast mating
David Younger, Stephanie Berger, David Baker, and Eric Klavins
To learn more about our technology or inquire about partnership opportunities, please get in touch.
Contact Us